Customer Data Analysis in Finance: Strategies and Insights
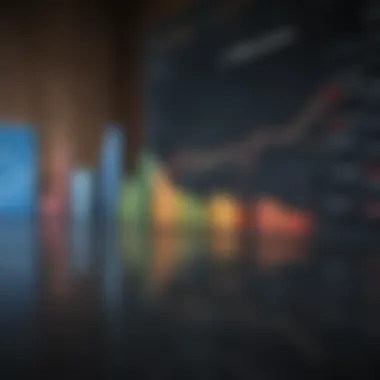
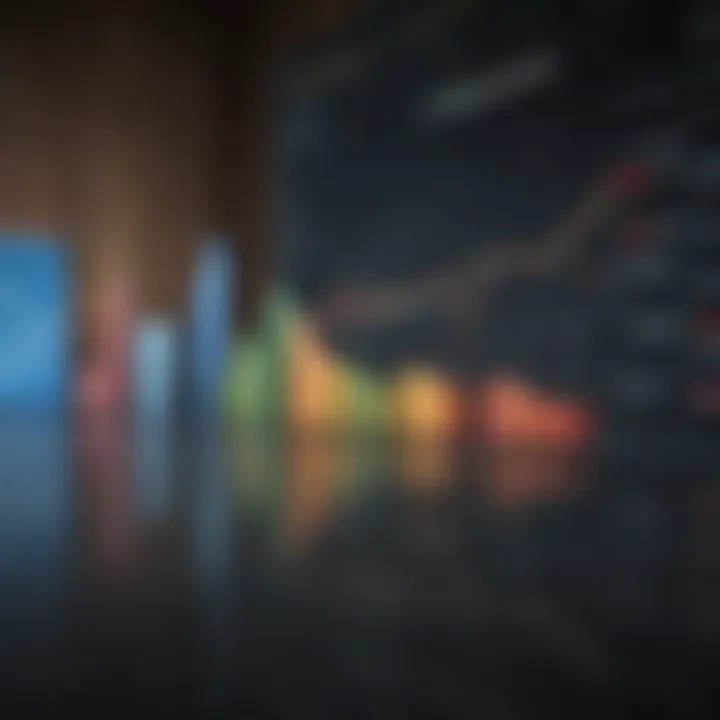
Intro
In the financial landscape today, customer data analysis stands as a pillar that supports strategic decision-making. Not only does it allow businesses to refine their services or products, but also it empowers investors to make informed choices based on comprehensive insights gleaned from client behavior. With the rapid evolution of technology and digitized platforms, the significance of analyzing customer data cannot be overstated. Both seasoned investors and new entrants benefit from understanding how data influences their financial strategies and enhances their competitive edge.
The figure of customer data is substantial. It encompasses behaviors, preferences, and demographics, cooking up a rich stew of insights that can influence not only marketing campaigns but also investment strategies. As we dive into this article, we'll tackle several core components: the fundamental concepts that define customer data analysis, effective strategies for investing based on these insights and ethical considerations that cannot be ignored.
Maximizing the potential of customer data isn't merely about collecting it; it’s about distilling valuable information that can impact financial decisions. This involves segmentation, profiling, and adopting a strategy that aligns with an investor's unique objectives. Moreover, the article will provide robust methodologies, tools, and frameworks that investors can employ to harness customer data more effectively.
Now, let’s unravel the key terms associated with this vast domain.
Prelude to Customer Data Analysis
In today's rapidly evolving financial landscape, the significance of analyzing customer data cannot be overstated. Financial decision-making, which has historically relied on broad market indicators or anecdotal evidence, is now being transformed by granular insights derived from detailed customer information. Any savvy investor recognizes that understanding individual customer behaviors, preferences, and buying patterns is invaluable.
By harnessing this knowledge, financial institutions can tailor their strategies to better align with the needs of their clientele, enhancing both profitability and customer satisfaction. This section aims to unwrap the layers of customer data analysis and its implications for financial entities, effectively acting as a compass for navigating the complexities of modern finance.
The journey into customer data analysis is not just about crunching numbers; it's about uncovering the hidden gems within the data, which can drive more informed decisions. Whether it's through identifying emerging trends or optimizing customer interactions, the ability to interpret customer data can confer a significant competitive advantage.
Importance of Customer Data in Finance
The role of customer data in finance is akin to the heartbeat of a thriving economy. It offers insights that can make or break investment strategies. Why? Because understanding customer needs enables financial firms to create products and services that truly resonate.
Take, for instance, credit scoring. Without robust customer data, determining creditworthiness would be akin to shooting in the dark. Every data point—from payment histories to spending habits—marks a step towards accurate assessments. Furthermore, in a world where personalization is king, tailoring communication and product offerings based on data insights enhances customer loyalty and retention.
The implications are profound.
- Higher profits: Targeted campaigns yield better results and higher conversion rates.
- Reduced risk: Proper data analysis can reveal potential defaults before they happen.
- Enhanced customer experience: Personalized interactions foster satisfaction and build trust.
Thus, customer data stands as a cornerstone of strategic financial planning.
Overview of Data Analysis Techniques
When it comes to dissecting customer data, a myriad of techniques are at one's disposal. Choosing the right method depends on the goals and the nature of the data itself. Various approaches can illuminate distinct facets of customer behavior.
- Descriptive Analysis: This technique provides a snapshot of past events, encapsulating historical customer interactions. For example, sales reports from a previous quarter can highlight what worked and what didn’t.
- Diagnostic Analysis: This goes a step further, attempting to answer the ‘why’ behind past customer behaviors. By applying this method, financial analysts can investigate, say, a sudden drop in customer account openings.
- Predictive Analysis: Leveraging statistical models, predictive analysis forecasts future trends based on historical data. Imagine trying to anticipate a market downturn by examining customer withdrawal patterns; this tool could be indispensable.
- Prescriptive Analysis: Now we’re discussing actionable insights. Once predictive insights are gathered, prescriptive analysis suggests specific actions to optimize outcomes. For instance, if data predicts a downturn, firms might choose to adjust investment strategies proactively.
- Sentiment Analysis: This modern technique harnesses social media and customer feedback to gauge public sentiment towards a financial entity. It reveals customer moods, preferences, and emerging concerns.
In sum, utilizing a diverse toolbox of data analysis techniques promotes a well-rounded perspective on customer activities, fortifying decision-making processes within financial realms.
"In the realm of finance, data isn't just important; it’s everything. The right analysis leads to smarter, more effective decisions."
By gathering accurate data and leveraging insightful analysis techniques, financial institutions can not only forecast but also sculpt the future of their customer interactions.
Data Collection Methods
Data collection methods are at the heart of understanding customer behavior and preferences in the financial sector. Without gathering the right kind of information, it's like trying to find your way in a fog without a map. Each method has its unique contribution, and knowing how to leverage them can make a world of difference in decision-making. The journey from raw data to actionable insights begins here.
Quantitative vs Qualitative Data
Quantitative data deals with numbers. It’s the hard facts that can make or break an analysis—how much customers are spending, how often they make purchases—and it can easily be analyzed using statistical tools. This type of data helps build a numerical foundation for understanding trends in customer behavior. On the flip side, qualitative data brings in color and depth. It encompasses opinions, motivations, and feelings, helping to paint a fuller picture of what makes customers tick.
The interplay between these two forms creates a more holistic view of customer behavior. For example, while quantitative data might show a dip in sales, qualitative insights through customer feedback can reveal whether it was due to a new competitor in town or customers simply feeling indifferent about the product. Therefore, both types are crucial—the straight-up numbers give you a structure while the qualitative bits offer context.
Sources of Customer Data
Understanding where to source customer data is just as important as knowing how to analyze it. Here, we’ll dissect three major sources of customer insights: surveys and questionnaires, customer transactions, and online interaction tracking. Each of these has its own flavor and brings different elements to the table.
Surveys and Questionnaires
Surveys and questionnaires are a tried-and-true method for gathering insights directly from customers. They serve as a direct line of communication, asking users specific questions about their experiences and preferences. What makes this method particularly appealing is its ability to reach a broad audience quickly. The key characteristic of these tools is their versatility—they can be tailored to gather a wide range of information, from product satisfaction to potential improvements. Customers often appreciate having the chance to voice their opinions.
A unique feature of surveys and questionnaires is their anonymity, which can encourage more honest responses. However, a downside could be the potential for low response rates, especially if participants don’t see immediate value in completing the survey. Still, when executed thoughtfully, they can yield insightful data that informs product development and marketing strategies.
Customer Transactions
Customer transactions are perhaps one of the most straightforward and valuable sources of customer data. Every purchase a customer makes tells a story about their preferences and habits. Notably, tracking transactions provides a wealth of transactional history that can reveal spending patterns, seasonal trends, and customer loyalty.
The key characteristic here is that this data is already collected through operational processes, making it relatively easy to access and analyze. A unique feature of customer transactions is their ability to connect past behaviors to future opportunities—for instance, understanding which products are frequently bought together can help in upselling efforts. However, one must be cautious about privacy concerns, ensuring that data is handled while adhering to regulations.
Online Interaction Tracking
With the rapid growth of digital channels, online interaction tracking has become increasingly significant. This method involves monitoring how customers engage with websites, social media, and other online platforms. It provides intrinsic details like page views, click patterns, and conversion rates.
The key characteristic of this tracking method is that it allows for real-time insights into customer behavior; businesses can adapt their strategies almost instantly based on current user interaction. A unique feature is the segmentation of visitors, enabling the differentiation between first-time visitors and returning customers. Yet, a disadvantage lies in the complexity of analyzing vast amounts of data, and there's always the risk of overwhelming insights that could lead to analysis paralysis.
Data Analysis Frameworks
In the realm of finance, analyzing customer data isn’t just beneficial — it’s imperative for making well-informed decisions. Data analysis frameworks provide a structured approach to examining complex datasets, allowing financial analysts to sift through numbers, trends, and patterns effectively. With the financial landscape becoming increasingly competitive, having a robust framework in place can markedly enhance strategic planning and operational efficiency.
When we talk about data analysis frameworks, we're often referring to methodologies that underpin statistical analysis and predictive models. These frameworks not only simplify data interpretation but also guide how financial institutions can derive actionable insights from customer behavior. Without a clear framework, data can be as confusing as a maze, leading analysts down blind alleys when it comes to decision-making.
Some benefits of implementing a solid data analysis framework include:
- Consistency: Having defined protocols ensures all analysts are on the same page, adhering to similar standards in evaluating data.
- Efficiency: With clear procedures, the time taken to analyze data is reduced, which is critical in a fast-paced financial environment.
- Insight Generation: A framework enhances the ability to extract meaningful insights that can influence strategies, from tailored marketing to risk assessment.
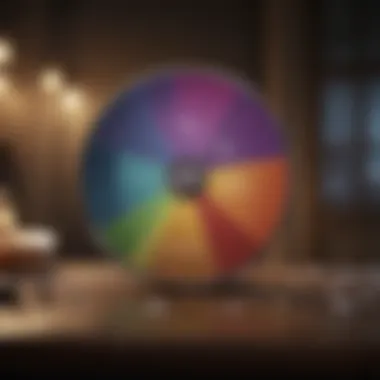
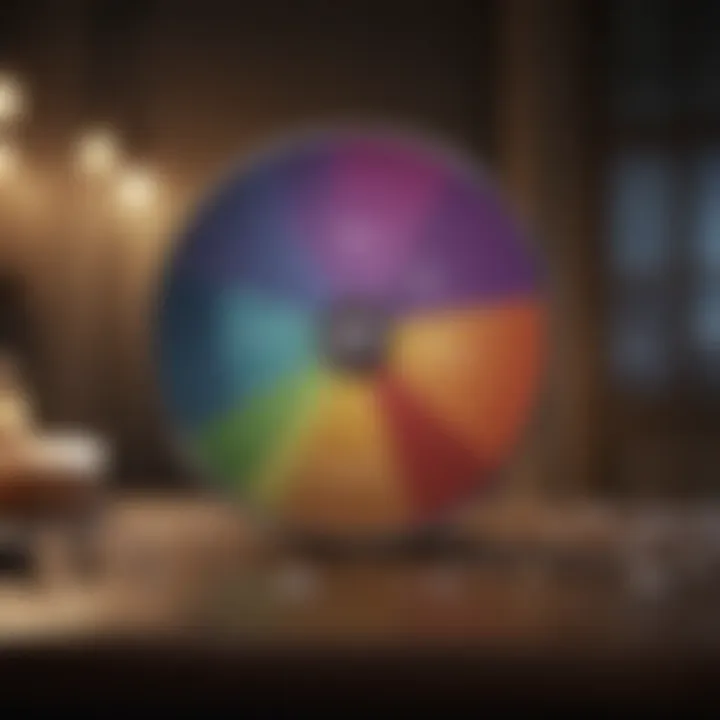
The critical considerations are ensuring the chosen framework becomes an integral part of the analytical culture within a financial institution. Data analysis shouldn't just be about crunching numbers; it should lead to a deeper understanding of customer needs and behaviors.
When establishing these frameworks, it’s essential to remain agile. The world of finance is akin to sailing a ship, one must adjust the sails according to shifting winds. Thus, continuously refining your frameworks based on feedback and new data is paramount.
Statistical Analysis Techniques
Statistical analysis techniques are foundational tools within any data analysis framework. They enable financial analysts to decode data and generate insights that may not be immediately evident. When applied correctly, statistical techniques offer a numerical lens through which patterns can emerge, paving the way for informed decision-making.
Common statistical techniques include:
- Descriptive Statistics: This provides summaries about the sample and measures such as means and standard deviations. Such insights can offer a quick snapshot of customer behavior.
- Inferential Statistics: This extends beyond the immediate data, allowing conclusions to be drawn about a larger population based on sample data. Hypothesis testing is a cornerstone here, helping analysts to infer trends across customer segments.
- Regression Analysis: By establishing the relationships among different variables, regression can help determine how various factors impact financial outcomes, like sales figures influenced by customer demographics.
Utilizing statistical techniques in an organized manner ensures that every piece of analyzed data contributes to a larger narrative about customer behavior.
Predictive Analytics Models
Predictive analytics models are like crystal balls for financial analysts. They utilize historical data to forecast future trends, helping financial institutions plan effectively for potential outcomes. The power of these models lies in their ability to transform raw data into predictions with substantial accuracy, impacting everything from customer targeting to risk management.
Key aspects of predictive analytics models include:
- Machine Learning Algorithms: These can analyze vast amounts of data and identify patterns that are often missed by human analysis. Techniques such as decision trees or neural networks can provide valuable insights into customer behavior and financial forecasting.
- Time Series Analysis: This method focuses on data points collected over time. It is particularly useful for tracking trends in customer behavior across various seasons or economic cycles, allowing institutions to adjust strategies accordingly.
- Risk Assessment Models: Understanding potential risks is essential in finance. Predictive modeling aids in assessing risk levels associated with specific customer segments, facilitating more informed lending decisions or investment strategies.
Incorporating predictive analytics into data frameworks allows organizations to stay ahead of the curve, tailoring approaches to meet anticipated customer needs.
"Data, when processed thoughtfully, can offer a treasure trove of insights that guide us towards the future of finance."
The structured approach of a good data analysis framework, combined with statistical and predictive techniques, ultimately empowers financial institutions to harness customer data effectively. Understanding these concepts is not just an option; it’s a necessity for any financial professional looking to thrive in a data-driven world.
Customer Segmentation and Profiling
Customer segmentation and profiling take center stage in the art of data analysis, particularly when it comes to financial decision-making. By breaking down the market into distinct groups, organizations can tailor their strategies to meet the specific needs of their customers. This is more than just a nice-to-have; it's a necessity in today’s competitive landscape. The financial sector thrives on understanding intricacies in customer behaviors, preferences, and demographics. It’s the difference between casting a wide net and fishing in the right pond.
The benefits of effective segmentation are manifold. For one, it allows for more personalized marketing approaches, which can significantly elevate customer engagement. Additionally, the segmentation can lead to enhanced product offerings, aligning them with what customers really want. When financial institutions target finely segmented groups, they act with surgical precision, improving both conversion rates and customer satisfaction. It drives not only sales but also loyalty, as customers feel recognized and valued in ways that broad campaigns often miss.
However, effective customer segmentation is not without its challenges. Companies must weigh the ethical considerations of handling personal data carefully. Privacy concerns can lead to customer distrust if data collection isn’t transparent. Thus, a balance must be struck between leveraging data for business benefits and safeguarding customer privacy.
Defining Segmentation Criteria
When it comes to creating segments, it’s crucial to define clear and actionable criteria. These criteria serve as the foundation upon which the entire segmentation strategy is built. Common parameters include:
- Geographic Location: Urban versus rural can yield vastly different customer behaviors.
- Behavioral Data: Understanding how customers interact with financial products can lead to better targeting.
- Financial Goals: Different customers have different objectives – from wealth accumulation to basic savings.
"The key to successful segmentation lies not just in the data collected, but how effectively that data translates into actionable insights."
Criteria need to be precise yet flexible enough to adapt as market conditions evolve. For instance, in the face of economic changes, segments based on spending behaviors may need rapid re-evaluation.
Use of Demographics in Segmentation
Utilizing demographics effectively is a cornerstone of customer segmentation. Age, income level, education, and occupation can reveal volumes about customer preferences and behavior. For instance, millennials might prioritize mobile app usability and personalized financial advice more than older generations. In contrast, baby boomers might lean towards more traditional investment options.
Demographics can also assist in creating customer personas, which offer a snapshot of your ideal customer types, aiding organizations in crafting focused marketing campaigns.
- Age Groups: Tailoring products for various age brackets can influence marketing messages profoundly.
- Income Levels: Offering different products, like credit loans with varied limits based on income, attracts diverse customers.
- Education and Occupation: Certain financial products may cater specifically to professionals in specialized fields.
Understanding these dynamics is not merely academic; it’s practical advice in designing services and communications that resonate well with target audiences. In a nutshell, leveraging demographics provides actionable insights essential for crafting strategies that are not only relevant but also compelling.
Visualizing Data Insights
Visualizing data insights plays a pivotal role in understanding customer behaviors and trends, especially in the financial sector. In a world where raw data can be overwhelming, turning complex datasets into intuitive visual formats allows stakeholders to grasp key patterns and make informed decisions quickly. Effective visualization not only illuminates significant insights but also helps in identifying anomalies and highlighting opportunities for growth.
Key benefits of employing visualization techniques include improving communication of insights, enhancing retention of information, and fostering a collaborative environment where discussions around customer data are richer and more informed. A well-crafted visual can tell a story that raw numbers alone fail to convey — it’s about translating data into actionable intelligence.
Tools for Data Visualization
Selecting the right tools can make a world of difference when it comes to data visualization. Here are some of the most effective tools frequently used in the finance world:
- Tableau: Known for its ability to create interactive and shareable dashboards, Tableau allows users to connect different data sources and analyze wealth data in an engaging manner.
- Microsoft Power BI: A powerful analytic tool that integrates seamlessly with Excel, Power BI enables users to visualize data intuitively, with ready-made templates suitable for financial analyses.
- Google Data Studio: This free tool is excellent for creating customizable reports. It allows real-time data connection, which is crucial in the fast-paced financial environment.
- QlikView: A staple in advanced analytics, QlikView offers associative data modeling and powerful visualization options that can help in deep-diving customer behavior.`
These tools not only enhance the aesthetic of presentations but also facilitate deeper insights through interactivity.
Best Practices in Presenting Data
When it comes to presenting data insights, following best practices ensures that the intended message lands effectively. Consider these practices:
- Know Your Audience: Tailor visuals for the specific audience, understanding their level of familiarity with the data.
- Simplify and Focus: Avoid clutter. Each graphic should have a clear purpose. Utilize whitespace to allow visuals to breathe.
- Choose the Right Type of Visualization: Line graphs work well for trends over time, whereas bar graphs can effectively compare quantities. Select the format that best tells your data's story.
- Label Clearly: Ensure that every axis, legend, and data point is clearly labeled to avoid confusion.
- Use Color Strategically: Colors should enhance comprehension rather than distract. Employ a consistent color scheme and utilize contrasting colors for emphasis.
- Tell a Story: Frame the data in a narrative context, guiding the viewer through the insights and highlighting key takeaways.
"A picture is worth a thousand words," and in the realm of data, a well-crafted visualization can significantly affect understanding and decision-making.
By following these practices, financial enthusiasts and individual investors can ensure their data insights are not just seen, but understood and utilized for effective financial decision-making.
Implementing Data-Driven Strategies
In today’s fast-paced financial landscape, adopting a data-driven approach is no longer optional; it is essential for those looking to gain a competitive edge. Implementing data-driven strategies allows financial institutions and individual investors to leverage insights derived from customer data to make more informed decisions. This practice not only enhances the effectiveness of marketing efforts but also significantly elevates the overall customer experience.
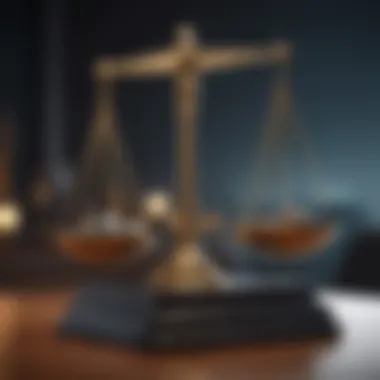
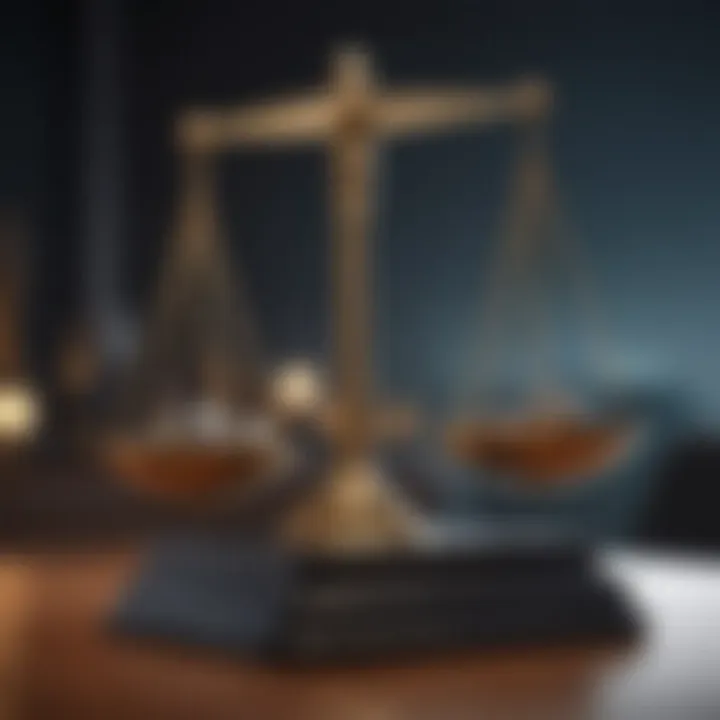
Understanding customer behavior, preferences, and trends can help organizations tailor their messaging and offerings in a way that resonates distinctly with different segments of the market. Not only does this approach improve customer engagement, but it also boosts satisfaction and loyalty. When investors use data to drive strategy, they embrace a more scientific method of decision-making, minimizing guesswork and relying on evidence.
Tailoring Marketing Efforts
A tailored marketing effort means honing in on specific customer segments and creating initiatives that speak to their unique needs. This can involve everything from personalized email campaigns to special promotions designed for targeted groups. Data analysis provides invaluable insights into who the customers are, what they want, and how best to reach them.
Using advanced segmentation techniques enables financial firms to categorize customers based on various criteria, such as demographics, behavioral data, and transaction history. Here are some benefits:
- Enhanced Relevance: Tailored messaging means that customers receive information that is pertinent to their financial objectives, leading to increased open rates and engagement.
- Improved ROI: By directing marketing resources towards the most promising segments, firms can obtain a higher return on their marketing investments.
- Real-time Modifications: With continuous data analysis, marketing strategies can be adjusted on the fly based on current trends and customer feedback.
It's vital to mention that personalization doesn’t only amplify engagement; it also fosters trust. For instance, when a financial service provider remembers a customer's preferences and comments, it shows that the business values the relationship beyond a mere transaction, which may prompt customers to be more loyal.
Enhancing Customer Experience
Enhancing the customer experience through data-driven strategies is about creating a seamless and rewarding journey for customers as they interact with financial services. This involves not just understanding where customers might face hurdles but also anticipating their future needs.
Key considerations in enhancing the customer experience include:
- Personalized Touchpoints: Utilizing data insights to create personalized recommendations or reminders can make customers feel valued and understood. For example, if a customer usually invests in technology stocks, a tailored notification about new opportunities in that sector would likely catch their interest.
- Streamlined Processes: Data analysis can reveal inefficiencies in customer service. By addressing these issues, like shortening wait times or simplifying onboarding processes, customer satisfaction can surge.
- Feedback Loops: Engaging customers in conversations and encouraging their feedback on products or services is crucial. This could be through follow-up surveys, which not only provide fresh insights but also make customers feel involved in the improvement process.
"A happy customer will tell a friend; an unhappy customer will tell the world."
By fostering an environment where customer voices are heard, businesses can rapidly adapt and innovate. Ultimately, enhancing the customer experience leads to longer-term relationships and brand loyalty, crucial elements in the financial services realm.
Embracing data-driven strategies doesn’t simply modify how financial firms operate; it transforms their very existence in the marketplace. As technology evolves and data becomes even more intricate, those who grasp these strategies early will be better positioned to reap their benefits.
Ethical Considerations in Data Analysis
In today’s fast-paced world, where data seems to be the lifeblood of businesses, understanding the ethical considerations behind analyzing customer data is paramount. These considerations serve as the bedrock for a responsible approach to data utilization in the financial sector, nurturing trust between companies and customers. It’s essential to strike a balance between leveraging data for strategic advantage and maintaining respect for customers’ privacy and rights.
Data Privacy Regulations
Financial institutions are no strangers to regulations regarding data privacy. With laws like the General Data Protection Regulation (GDPR) in Europe and the California Consumer Privacy Act (CCPA) in the United States, organizations must tread carefully when handling customer data. These regulations not only mandate the proper collection and storage of data but also require transparency about how the data will be used.
- Consent is Key: Customers should give explicit consent before their data is collected. This means clear communication about what data is being gathered and for what purpose.
- Right to Access: Customers have the right to access their data, enabling them to see what information is held and ensuring that it’s accurate and relevant.
- Data Breach Protocols: Organizations ought to have a plan in place to address data breaches. Should a breach occur, notifying affected customers in a timely fashion is crucial to maintaining trust.
Understanding and adhering to these regulations not only avoids legal pitfalls for organizations but also fosters a culture of respect and integrity around customer data.
Transparency in Data Usage
Transparency is the secret sauce that fosters trust in the customer-organization relationship. It involves open communication about how customer data is being collected, analyzed, and used. Financial institutions that embrace transparency often find themselves reaping significant rewards.
- Building Trust: When customers know how their data is being used, they are more likely to engage with a company. This translates to better customer retention and loyalty.
- Clarity of Purpose: Businesses should convey exactly why they collect customer data. Are they using it to enhance the customer experience, improve service, or tailor marketing strategies? Clear communication of the purpose helps in driving customer satisfaction.
- Providing Opt-Out Options: Offering customers the ability to opt-out of data sharing or usage can be a powerful move. It shows respect for individual preferences, nurturing a more favorable image of the organization.
Transparency not only facilitates compliance with existing regulations, but it also sets the groundwork for ethical data practices that align with the evolving expectations of customers.
Overall, incorporating these ethical considerations into data analysis strategies can lead to a more respectful, trusting relationship between financial institutions and their customers, ultimately paving the way for more informed and ethical financial decision-making.
Technology's Role in Data Analysis
In the fast-paced world of finance, technology stands as a pivotal element in the analysis of customer data. Not only does it enhance the efficiency and accuracy of data processing, but it also enables businesses to uncover deep insights that were previously obscured by overwhelming volumes of information. Without effective technological tools, the daunting task of extracting actionable intelligence from customer data can feel akin to searching for a needle in a haystack.
The integration of advanced technology plays an invaluable role in several aspects of data analysis. For instance, computational power allows analysts to run intricate algorithms on vast data sets in a matter of minutes, a task that would take humans a considerable amount of time. Moreover, tools designed for data visualization enable stakeholders to digest complex information quickly through intuitive graphics and dashboards, facilitating faster decision-making.
Benefits of Technology in Data Analysis
- Speed and Efficiency: Automated data processing tools reduce manual work, enabling quicker insights.
- Accuracy: Reduces human error by relying on algorithms and programmed analyses.
- Scalability: As data grows, technology allows scaling operations without compromising performance.
- Customization: Advanced analytics tools can be tailored to meet specific business needs, enhancing the relevance of insights derived.
Considerations Regarding Technology's Role
While technology undoubtedly propels data analysis forward, it isn’t without its challenges. Relying solely on technology may lead businesses to overlook the qualitative aspects of data, which can provide context to numbers. Furthermore, employing sophisticated tools requires skilled personnel who understand not just how to operate these technologies, but also how to interpret the results meaningfully.
Emerging Technologies in Data Analysis
Emerging technologies are revolutionizing the landscape of data analysis in finance. These innovations serve as catalysts for transformation, enabling organizations to tap into new sources of value. Technologies like blockchain, advanced data storage solutions, and high-performance computing are paving the way for modern data analysis practices.
- Blockchain Technology: Ensures secure, transparent transactions while enabling real-time data sharing and tracking.
- Cloud Computing: Offers flexible data storage and accessibility, allowing financial organizations to scale operations without incurring heavy infrastructure costs.
- Advanced Data Warehousing: Facilitate the integration of structured and unstructured data from diverse sources, enriching the analysis with diverse data sets.
Impacts on Financial Decision-Making
The effect of these technologies extends beyond mere efficiency; they empower financial firms to be proactive rather than reactive. For instance, the enhanced capabilities in predictive analytics allow organizations to anticipate customer needs before they arise, effectively turning data into a strategic asset.
Artificial Intelligence and Machine Learning
Artificial intelligence (AI) and machine learning (ML) are perhaps the most talked-about advancements in data analysis today. The core advantage of these technologies lies in their ability to learn from data patterns and trends without being explicitly programmed for each task, which is a game-changer for the finance sector.
Applications in Financial Services
- Fraud Detection: AI systems analyze transaction patterns to identify anomalies indicative of fraud, increasing security.
- Risk Management: Machine learning algorithms can predict potential risks based on historical data, enhancing strategic decision-making.
- Customer Insights: By analyzing customer behavior and preferences, AI-driven analytics provide tailored recommendations that foster deeper customer loyalty.
"The future of finance is no longer in traditional methods, but in leveraging artificial intelligence to make data-driven decisions that have the power to change the game."
In essence, the synergy between technology, AI, and machine learning fosters a more nuanced understanding of customer data, positioning financial institutions to respond sensitively and effectively to changing market dynamics. As both the technology and methodologies continue to evolve, staying current is not just advisable, but essential for sustained success in financial analysis.
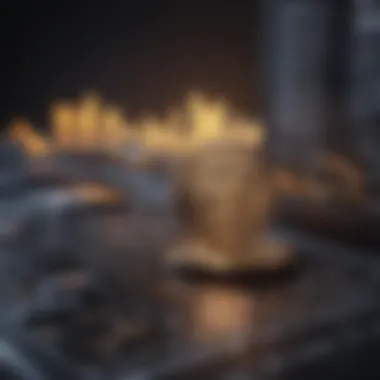
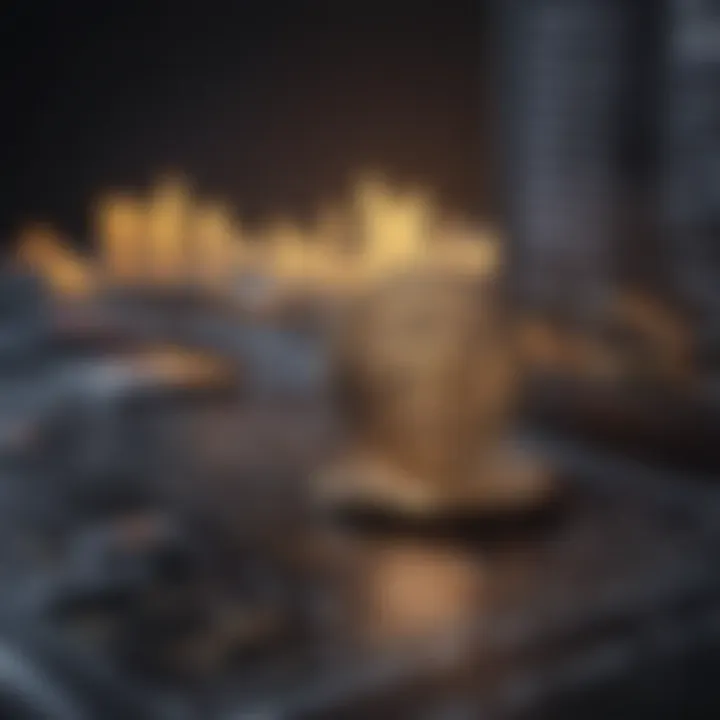
Challenges in Analyzing Customer Data
Analyzing customer data is like trying to put together a jigsaw puzzle with a few pieces missing—always a challenging task. The financial sector thrives on accurate insights derived from data, but various challenges can muddy the waters. Overcoming these hurdles not only enhances our understanding of customer behavior but also refines decision-making processes that impact financial outcomes. Let’s explore some distinct challenges that practitioners in this field must navigate.
Data Quality Issues
First and foremost, the reliability of any analysis hinges on the quality of data collected. Poor data quality can lead to skewed results and misguided decisions. A classic example: imagine working with customer data sets cluttered with duplicate entries. This can happen when transactions from the same customer are recorded multiple times, resulting in an inflated view of customer activity. To mitigate this, organizations must adopt stringent data validation practices.
Key aspects of data quality include:
- Accuracy: Is the data correct? Did a customer indeed interact with the service or product as recorded?
- Consistency: Are records uniform across all sources? Misaligned data entries can present a façade of trends that don’t really exist.
- Completeness: Is all vital information captured? Leaving fields blank can distort analysis, leadingone to unfinished insights.
- Timeliness: Data must be current to ensure relevance; outdated information can misguide financial strategies.
By focusing on these aspects, businesses can bolster data credibility, leading to more sound analysis and reporting. The "garbage in, garbage out" idiom rings particularly true in financial decision-making; one must start with solid data.
Integrating Diverse Data Sources
The financial landscape today is brimming with data coming from various sources—customer transactions, social media interactions, feedback forms, and much more. This diversity, however, brings its own set of complications. Integrating this data into a coherent form for analysis is no walk in the park.
Often, data resides in silos across departments; sales might have customer purchase data, while marketing archives lead generation data. Bridging these silos is crucial. Here’s why:
- Holistic View of Customers: Combining data from different channels provides a 360-degree view of customer interactions, enhancing understanding of behaviors and preferences.
- Cross-Department Collaboration: When various functions share insights, it can vastly improve marketing strategies, customer relationship management, and ultimately, financial performance.
- Better Predictions: Tapping into multiple data sources allows companies to build more robust predictive analytics models. For instance, examining how marketing campaigns affect purchasing patterns can lead to sharper forecasts and strategies.
Techniques such as data warehousing and the use of cloud platforms can facilitate this integration. These tools hold the promise of centralizing customer information, making life just a bit easier for analysts and decision-makers.
"Without a well-integrated data architecture, any analysis is like building a castle on quicksand."
By addressing issues related to data quality and source integration, financial practitioners armed with the right insights can navigate the complex waters of customer data analysis more effectively.
Case Studies: Success in Data Analysis
Case studies are often the cherry on top when it comes to understanding how customer data analysis translates into real-world success. They give us a front-row seat to the strategies employed by industry leaders and allow us to dissect their methods, uncovering the nuances of decision-making based on data insights. This section will delve into specific cases that illustrate how effective data analysis can drive significant improvements in engagement and operational efficiency within the financial sector.
Understanding the benefits of these case studies is root in practical application; they offer a roadmap for organizations looking to leverage customer data. Not only can they pinpoint what works and what doesn’t, but they also shed light on larger trends emerging in the market. For financial enthusiasts and individual investors, this analysis is not merely academic; it’s about grasping the trends that could affect their portfolios and investment choices.
Retail Engagement through Data
The world of retail is a remarkable tableau for examining the impact of data analysis. One notable case is the strategies implemented by Target Corporation, which combined demographic analysis with purchasing behaviors to create highly personalized marketing campaigns. They discovered that by analyzing historical customer data, they could predict life events, like pregnancy, and tailor promotions accordingly.
- Segmenting Target Audience: Through the collection of purchase data, Target identified trends that led to the creation of customized marketing strategies aimed at specific demographics, ultimately increasing customer engagement.
- Impact of Predictive Analytics: This predictive approach enabled Target to improve its marketing efficacy, resulting in a noticeable boost in sales. As they honed in on what resonates with their customers, their revenue surged significantly.
- Feedback Loop: More importantly, the constant evaluation of new data allowed them to refine their strategies further, moving beyond a one-size-fits-all approach to a more nuanced understanding of their customer base.
This case not only underscores the effectiveness of data analytics in achieving higher retail engagement but also reinforces the importance of understanding customer motivations and preferences.
Financial Services Optimization
In the realm of financial services, analyzing customer data has opened doors to optimization that were previously unimaginable. A well-documented example is JPMorgan Chase. They have incorporated advanced analytics to enhance their decision-making process significantly.
- Data Integration Across Platforms: By integrating various customer data from their banking operations, such as credit card usage and loan applications, they create comprehensive profiles that allow for better service offerings and customization.
- Risk Assessment: Furthermore, JPMorgan has deployed machine learning algorithms that sift through vast amounts of transaction data to identify patterns of fraud and customer behavior that may signal credit risks. This technological adoption has minimized potential losses and streamlined operations.
- Continuous Improvement: The key takeaway from JPMorgan's journey illustrates that data analysis isn’t just a one-time effort. It requires ongoing investment in systems and processes to continue refining and optimizing how financial services are delivered.
These case studies, be it from retail giants like Target or banking leaders like JPMorgan Chase, demonstrate the transformative power of customer data analysis. They serve as compelling proof that when organizations make informed, data-driven decisions, they not only meet the immediate needs of their customers but also position themselves at the forefront of their industries.
Future Trends in Customer Data Analysis
As we peer into the future of customer data analysis, it’s clear that this field is evolving rapidly. Understanding these changes is not merely an exercise in curiosity; it’s foundational for financial decision-making. Companies looking to stay competitive must adapt to shifting consumer expectations, technological advancements, and regulatory landscapes. The implications are vast, affecting everything from marketing strategies to customer service interactions.
Increased Personalization
In the realm of financial services, increased personalization is no longer a luxury; it's a necessity. Customers have come to expect tailored experiences that cater precisely to their needs, habits, and preferences. By leveraging advanced data analytics, businesses can uncover rich insights into customer behavior.
- Adapt familiar products: For instance, a bank might utilize transaction data to identify spending patterns, thereby recommending credit cards that align best with individual habits, such as travel or dining.
- Predictive modeling: Tools to predict future purchases and services can greatly enhance customer satisfaction. Imagine a scenario where an investment firm suggests fund options based on a client’s past investment behavior and risk tolerance.
This level of personalization not only fosters stronger customer loyalty but also drives increased sales. When customers feel understood, they are more likely to engage more deeply and remain with a provider longer.
The Growing Role of Big Data
Big data is, without a doubt, revolutionizing how financial institutions analyze customer information. The sheer volume, velocity, and variety of data now available is staggering, offering endless opportunities for those who know how to harness it.
- Data integration: In merging data from various sources, including social media, transactional data, and user engagement metrics, financial institutions can sculpt a comprehensive profile of their customers. This integration allows for strategies that are precisely targeted and highly effective.
- Advanced analytics: The sophistication of analytics tools is increasing. For instance, algorithms powered by machine learning can not only identify trends but can also forecast relevant outcomes with impressive accuracy.
With the right approach, businesses can turn challenges associated with big data into significant advantages. They can capitalize on opportunities that might have previously gone unnoticed, leading to better product development, improved market strategies, and ultimately, more informed decision-making.
"In the world of big data, it’s not just about having data; it's about how you use it to drive growth and innovation."
As we look ahead, the future trends of increased personalization and the growing role of big data will undeniably shape the landscape of customer data analysis within finance, intertwining with broader economic narratives and directly impacting stakeholders.
Employing these trends thoughtfully will empower financial firms to thrive in increasingly competitive markets.
Ending
In the rapidly evolving financial landscape, analyzing customer data isn’t just an optional strategy; it’s become a vital component of successful decision-making. The insights drawn from comprehensive customer data analysis can dictate not just the immediate strategies but also long-term business evolutions. With a solid grasp on customer behavior and preferences, businesses can effectively tailor their offerings, marketing campaigns, and overall engagement strategies, ensuring they meet the distinct needs of their audience.
Recap of Key Insights
- Customer Data Importance: The significance of customer data in finance cannot be overstated. It serves as the backbone for informed financial strategies, which help organizations navigate through uncertainties and seize emerging opportunities.
- Diverse Methods of Data Collection: Effective data analysis relies on a mix of qualitative and quantitative approaches. This variety allows for a more rounded understanding of customer behavior, leading to better predictive outcomes.
- Technological Integration: The rise of machine learning and artificial intelligence has opened new avenues for data analysis. Emerging technologies enable financial firms to process large volumes of data quickly and derive actionable insights.
- Ethical Risks: In the pursuit of customer insights, organizations must remain vigilant about data privacy and adhere to established regulations. Balancing proactive data usage with ethical considerations is crucial for sustaining customer trust.
- Future Trends: Looking ahead, trends like increased personalization and the growing role of big data are becoming central to customer data strategies. Financial services that embrace these trends are poised to gain a competitive edge.
Final Thoughts on Customer Data Analysis
As we conclude, it’s essential to recognize that the success of any financial strategy hinges on the ability to harness customer data effectively. It’s not merely about collecting information; it’s about interpreting that data to inform real-world decisions. This cyclic practice creates a feedback loop, whereby insights from customer interactions directly influence strategic financial decisions.
The continuous evolution of data analytics, paired with ethical best practices, will ensure that organizations remain adaptable and responsive to their customers’ needs. Ultimately, making data-driven decisions represents a commitment to value—both for the investor and the clientele. Therefore, honing skills in customer data analysis isn’t just an asset; it's a necessity for anyone serious about advancing in the financially driven world.